RAGing Against AI Hallucinations
Can this tech make AI more trustworthy?
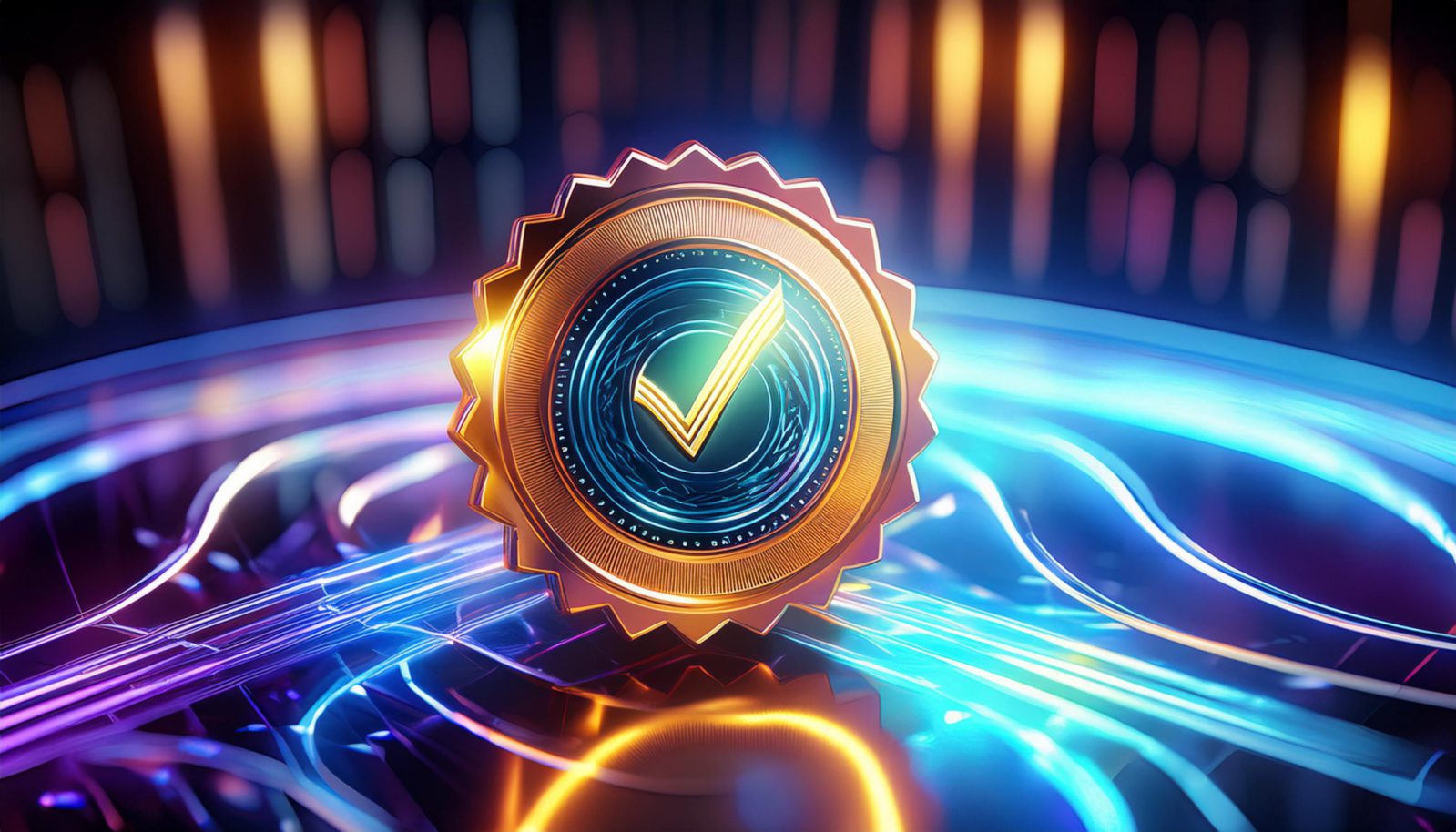
AI’s tendency to create fabricated information remains a major hurdle (and risk) against its continued adoption.
This is where Retrieval-Augmented Generation (RAG) comes in. This new technology has the potential to revolutionize how AI interacts with the world.
RAG works by allowing AI models to access and integrate real-world data during the generation process. Traditionally, AI relies solely on the information it’s trained on, which can lead to biases and factual inconsistencies. RAG, however, empowers AI to “fact-check” itself in real time, pulling from external sources like news articles, research papers, and even corporate databases (with permission) to ensure its responses are grounded in truth.
This integration of real-world data has exciting implications across various fields. A recent Stanford University study exploring AI legal assistants equipped with RAG capabilities demonstrated a significant reduction in factual errors compared to traditional large language models (LLMs). Similarly, an article by AdExchanger explores the potential for RAG to leverage first-party data, highlighting its ability to personalize experiences and improve campaign performance in marketing.
RAG holds immense promise, but researchers acknowledge it’s a work in progress.
Several studies highlight the need for further development to address potential limitations in RAG technology. Additionally, the quality and reliability of external data sources integrated into RAG systems remain a critical focus.
The evolution of RAG highlights a broader trend in AI development: the increasing importance of incorporating human oversight and real-world data. As AI continues to permeate our lives, ensuring its accuracy and reliability is paramount.